Data science connects the world by condensing previously dispersed information into small components. But, with all the talk about big data and how businesses use it, you might be wondering, "What types of data are we talking about?"
To begin, it's important to recognize that data is critical to business decisions. It is the lifeblood of any company and comes in a wide range of formats, from well-structured relational databases to your most recent Instagram post, or the recording of a meeting held a few weeks ago. The ability of an organization to obtain relevant data, evaluate it, and act on its findings is what determines its level of success. However, the amount and types of data available to businesses are ever-expanding. Secondly, all data isn't created equally. As a result, data generated by social media apps differ significantly from data generated by supply chain systems. In all its different formats, all of this data can be divided into two main categories: structured data and unstructured data, and the way this data is collected, processed, and analyzed depends on its format.
Before we get into the unique differences between structured and unstructured data, it's vital to understand that using versus does not imply that one is better than the other. Instead, it simply implies that organizations are debating whether to invest in unstructured data analytics and if it is conceivable to combine the two for better business intelligence.
What is Structured Data?
Structured data is most commonly classified as quantitative data, and it's the form of data with which most of us are familiar. It is the data in relational databases and spreadsheets that fits nicely into specified fields and columns. Examples of structured data include names, dates, addresses, credit card numbers, stock information, geo location, and more of the like.
Machine language and artificial intelligence tools can easily understand structured data because it is well-organized. For example, those working with relational databases can use a relational database management system to quickly input, search, and manipulate structured data.
It's crucial to remember that while this data may not appear relevant at first glance, it's crucial to remember that using analytic tools can reveal patterns and trends that can benefit the business. While structured data has effectively replaced paper-based systems for corporate intelligence for decades, more firms are turning to deconstruct unstructured data for future potential.
What are the Use Cases for Structured Data?
Structured data may be searched, analyzed, and understood by people and robots. Structured data has a clear schema and is organized. Structured data uses include:
- Financial Analysis and Reporting:
Financial reporting requires structured data. Financial institutions and accounting departments analyze transactions, balance sheets, income statements, and other financial records using structured data.
- Relational Databases:
Relational databases hold structured data in tables with well-defined entity relationships. This simplifies searching, indexing, and retrieval. Databases store consumer data, goods, financial transactions, and more.
- Customer relationship management (CRM):
CRM software creates datasets that show patterns and trends in customer behavior by processing structured data using analytical tools.
What is Unstructured Data?
Unstructured data is frequently classified as qualitative data since it cannot be handled or analyzed using traditional tools and methodologies. Examples of unstructured data include text files, emails, social media, websites, mobile data like geolocation or text messages, media files, satellite imagery, sensor data like traffic or weather, etc.
Because unstructured data lacks a pre-defined data model, it cannot be organized in relational databases, making it difficult to deconstruct. Non-relational or No SQL databases, on the other hand, are ideal for handling unstructured data. Unstructured data can also be managed by flowing into a data lake in its raw, unstructured form.
While organized data provides a bird's-eye view, unstructured data can provide a far more in-depth understanding of the subject. However, it's not easy to find the insights buried in unstructured data and to make an impact, complex analytics and a high level of technological competence are required. For many businesses, data analysis is a costly transition. Those who can use unstructured data, on the other hand, have a competitive advantage.
What are the Use Cases for Unstructured Data?
Unstructured data has no schema or format. Text, photos, videos, social media postings, emails, audio recordings, and other forms are common. Unstructured data has many useful uses despite its organizing and analytical limitations. Unstructured data uses include:
- Natural Language Processing (NLP):
Unstructured text data, such as emails, social media posts, customer reviews, and articles, can be processed using NLP techniques.
- Data mining:
Enables companies use unstructured data to understand customer behavior, product sentiment, and purchase trends in order to better serve their customers.
- Chatbots:
Text analysis is carried out by chatbots in order to direct customers' enquiries to the most relevant information sources.
Structured Vs Unstructured Data
There are some notable differences between structured and unstructured data that data workers should be aware of.
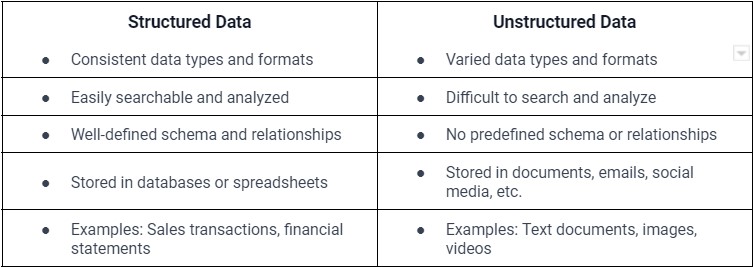
Artificial Intelligence and Machine Learning: The Next-Gen Game-Changing Tools
Analysts could search unstructured data using keywords and key phrases a few years ago and get a good idea of what was there. However, today unstructured data has grown to the point that data workers require advanced analytics and tools that run at computer speeds and learn from their actions and conclusions. As a result, new methods for analyzing unstructured data came into existence.
Artificial intelligence (AI) and machine learning (ML) are at the heart of most of these tools. Natural language processing (NLP), pattern recognition and classification, text mining methods, document relevance analytics, sentiment analysis, and filter-driven web harvesting are just a few examples. AI and ML play roles that are of utmost significance in operating, processing, and analyzing unstructured data.
Roles of AI and ML in Deriving From Unstructured Data
1. One of the most significant roles that Artificial Intelligence and Machine Learning play in analyzing unstructured data is overcoming the major challenges that have been concerning knowledge workers for decades in structured vs unstructured data include:
- Automating data workflow
- Standardizing unstructured data formats using traditional object-relational mapping techniques
- Complying governance
- Evaluating new technologies to understand human-generated data
- Increasing accessibility
2. Among the different sources of unstructured data, texts become of the most challenging kinds. This is so because one can never be absolutely sure of what they will receive, it’s never the same as shared before, and the most important reason is that it is in human language that has ambiguities and incomplete, inconsistent cases. However, AI and ML techniques like pattern recognition and sentiment analysis make it possible to not only understand but also make use of such complex unstructured data.
3. AI and ML run complicated analytics on unstructured data analytics and help:
- Identify data points from millions of unformulated data formats
- Categorize data and rectify errors and redundancies
- Study data relationships, conduct data modelling
- Implement data visualization
- Use digital imaging technologies and pattern recognition to interpret images and video content
- Implement Natural Language Processing (NLP) to obtain insights into human-generated, natural language unstructured texts and queries that traditional machine languages cannot comprehend
- Empower data analysts with greater automation, better efficiency, enhanced scalability, and high accuracy in processing and understanding unstructured data.
Organizations will improve existing products, increase the efficiency of internal processes, and enable informed decision-making by incorporating AI and ML and analyzing the insights obtained from structuring unstructured data. The structured data vs unstructured data debate is becoming increasingly important for businesses to consider.
Unstructured Data is the Future
For the foreseeable future, structured data will undoubtedly continue to play a role in most company activities. However, as data management improves and user data gets more complicated, the additional context added by unstructured data will become increasingly important. In addition, the capacity to store, query, and analyze data from any source will offer new possibilities, and it will be the place where businesses will find future success.
With Needl.ai, you can learn to unlock the hidden potential of unstructured data as we bring together category leaders in discrete apps, productivity tools, office suite platforms, communication apps, and workflow management tools that manage unstructured data and build uniform processing, collaboration, and information workflows. Moreover, we assist you in leveraging AI and ML to deal with unstructured data, streamline your workflow, and improve your experience.